MRI-Based Intratumoral and Peritumoral Radiomics for Preoperative Prediction of Glioma Grade: a multicenter study
Background: Accurate preoperative prediction of glioma is crucial for developing individualized treatment decisions and assessing prognosis.
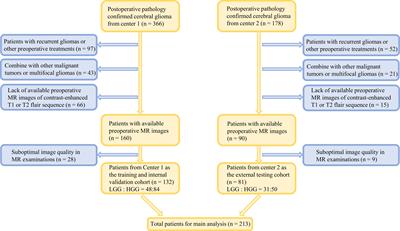
In this study, we aimed to establish and evaluate the value of integrated models by incorporating the intratumoral and peritumoral features from conventional MRI and clinical characteristics in the prediction of glioma grade. Methods: A total of 213 glioma patients from two centers were included in the retrospective analysis, among which, 132 patients were classified as the training cohort and internal validation set, and the remaining 81 patients were zoned as the independent external testing cohort. A total of 7728 features were extracted from MRI sequences and various volumes of interest (VOIs). After feature selection, 30 radiomic models depended on five sets of machine learning classifiers, different MRI sequences, and four different combinations of predictive feature sources, including features from the intratumoral region only, features from the peritumoral edema region only, features from the fusion area including intratumoral and peritumoral edema region (VOI-fusion), and features from the intratumoral region with the addition of features from peritumoral edema region (feature-fusion), were established to select the optimal model. A nomogram based on the clinical parameter and optimal radiomic model was constructed for predicting glioma grade in clinical practice.The intratumoral radiomic models based on contrast-enhanced T1-weighted and T2-flair sequences outperformed those based on a single MRI sequence. Moreover, the internal validation and independent external test underscored that the XGBoost machine learning classifier, incorporating features extracted from VOI-fusion, showed superior predictive efficiency in differentiating between low-grade gliomas (LGG) and high-grade gliomas (HGG), with an AUC of 0.805 in the external test.
The radiomic models of VOI-fusion yielded higher prediction efficiency than those of feature-fusion. Additionally, the developed nomogram presented an optimal predictive efficacy with an AUC of 0.825 in the testing cohort. Conclusions: This study systematically investigated the effect of intratumoral and peritumoral radiomics to predict glioma grading with conventional MRI.
The optimal model was the XGBoost classifier coupled radiomic model based on VOI-fusion.
The radiomic models that depended on VOI-fusion outperformed those that depended on feature-fusion, suggesting that peritumoral features should be rationally utilized in radiomic studies.
Read the full article at the original website
References: