Riemannian transfer learning based on log-Euclidean metric for EEG classification
Brain computer interfaces (BCI), which establish a direct interaction between the brain and the external device bypassing peripheral nerves, is one of the hot research areas.
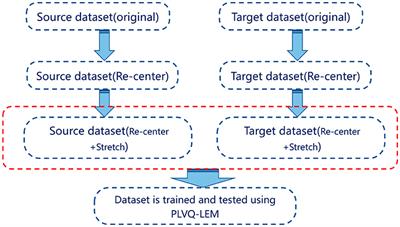
How to effectively convert brain intentions into instructions for controlling external devices in real-time remains a key issue that needs to be addressed in brain computer interfaces.
The Riemannian geometrybased methods have achieved competitive results in decoding EEG signals. However, current Riemannian classifiers tend to overlook changes in data distribution, resulting in degenerated classification performance in cross-session and/or cross subject scenarios. This paper proposes a brain signal decoding method based on Riemannian transfer learning, fully considering the drift of the data distribution. Two Riemannian transfer learning methods based log-Euclidean metric are developed, such that historical data (source domain) can be used to aid the training of the Riemannian decoder for the current task, or data from other subjects can be used to boost the training of the decoder for the target subject.
The proposed methods were verified on BCI competition III IIIa and IV 2a datasets. Compared with the baseline that without transfer learning, the proposed algorithm demonstrates superior classification performance. In contrast to the Riemann transfer learning method based on the affine invariant Riemannian metric, the proposed method obtained comparable classification performance, but is much more computationally efficient. With the help the proposed transfer learning, the Riemannian classifier obtained competitive performance to existing methods in the literature.
Read the full article at the original website
References: